[Editor’s Note: Today’s post poses four central questions to our Mad Scientist community of action regarding bias in machine learning and the associated ramifications for artificial intelligence, autonomy, lethality, and decision-making on future warfighting.]
“We thought that we had the answers, it was the questions we had wrong” – Bono, U2
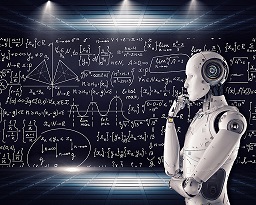
As machine learning and deep learning algorithms become more commonplace, it is clear that the utopian ideal of a bias-neutral Artificial Intelligence (AI) is exactly just that. These algorithms have underlying biases embedded in their coding, imparted by their human programmers (either consciously or unconsciously). These algorithms can develop further biases during the machine learning and training process. Dr. Tolga Bolukbasi, Boston University, recently described algorithms as not being capable of distinguishing right from wrong, unlike humans that can judge their actions, even when they act against ethical norms. For algorithms, data is the ultimate determining factor.
Realizing that algorithms supporting future Intelligence, Surveillance, and Reconnaissance (ISR) networks and Commander’s decision support aids will have inherent biases — what is the impact on future warfighting? This question is exceptionally relevant as Soldiers and Leaders consider the influence of biases in man-machine relationships, and their potential ramifications on the battlefield, especially with regard to the rules of engagement (i.e., mission execution and combat efficiency versus the proportional use of force and minimizing civilian casualties and collateral damage).
“It is difficult to make predictions, particularly about the future.” This quote has been attributed to anyone ranging from Mark Twain to Niels Bohr to Yogi Berra. Point prediction is a sucker’s bet. However, asking the right questions about biases in AI is incredibly important.
The Mad Scientist Initiative has developed a series of questions to help frame the discussion regarding what biases we are willing to accept and in what cases they will be acceptable. Feel free to share your observations and questions in the comments section of this blog post (below) or email them to us at: usarmy.jble.tradoc.mbx.army-mad-scientist@mail.mil.
1) What types of bias are we willing to accept? Will a so-called cognitive bias that forgoes a logical, deliberative process be allowable? What about a programming bias that is discriminative towards any specific gender(s), ethnicity(ies), race(s), or even age(s)?
2) In what types of systems will we accept biases? Will machine learning applications in supposedly non-lethal warfighting functions like sustainment, protection, and intelligence be given more leeway with regards to bias?
3) Will the biases in machine learning programming and algorithms be more apparent and/or outweigh the inherent biases of humans-in-the-loop? How will perceived biases affect trust and reliance on machine learning applications?
4) At what point will the pace of innovation and introduction of this technology on the battlefield by our adversaries cause us to forego concerns of bias and rapidly field systems to gain a decisive Observe, Orient, Decide, and Act (OODA) loop and combat speed advantage on the Hyperactive Battlefield?
For additional information impacting on this important discussion, please see the following:
• An Appropriate Level of Trust… blog post
• Ethical Dilemmas of Future Warfare blog post
• Ethics and the Future of War panel discussion video
Thanks to Kahneman and Tversky’s work we are well aware of the biases that bound human cognition, but we don’t always appreciate that any computational system is bounded. Gigerenzer provides an important balance to this, emphasizing that the heuristics that humans use are typically better calibrated to the practical constraints of complex problems than the quantitative logical or economic ideals. See my Perspicacity Blog for more detailed discussion of these ideas: https://blogs.wright.edu/learn/johnflach/2018/03/22/cognitive-systems-engineering-capabilities-and-limitations/
FWIW-it might be useful to discriminate between “cultural bias” and “bias”. Many systemic errors in AI output could potentially by categorized as some form of bias. Is the article suggesting accounting for any systemic error as bias, or only culturally induced biases, both, or something else. As background, in “Critical Thinking: An Introduction to Reasoning Well” (by Watson & Arp) they define “bias” as: “A systemic inaccuracy in data due to the characteristics of the process employed in the creation, collection, manipulation, and presentation of data, or due to faulty sample design of the estimating technique”. They define “cultural bias” as: “the phenomenon of interpreting and judging phenomena by standards inherent to one’s own culture. For example, wording questions on a test or survey so that only native speakers or members of a certain economic class can respond accurately.”
Except for natural laws and pure mathematics, bias is an unmistakable characteristic of a system. One notable difference with machine learning is the ability to quantify bias and reduce a series of subjective concerns to numerical observation. The history of the learning process, the outcome of training and gaming, and the probabilities of actions for specific outcomes provide the ability to virtually measure the behavior of the machine at machine time scales. Any and all bias should be welcome and in the requirements phase a determination of relevance and risk should be made to provide history, context, independent indicators and a means or predicting outcomes from learning systems as they evolve. This last phase, evolution, means the system continuously learns, modifies and adapts and so the parameters need to be at a level and granularity to measure bias against a subjective standard and that standard should be a clear parametric indicator.
A general set of parameters may not provide sufficient indication of bias, stability or risk for learning systems. An indication of iterative assessment, cycles or churn may provide some ability to measure problem complexity/ambiguity or conflict. Algorithms used in modern weapon systems apply the RoE established in the weapon requirement cycle and evaluated through T&E. Some systems may require behavioral measurements on a continuous T&E system – so an AI system or algorithmic monitor might act as the guide and possibly as mentor and analyst to avoid unintended actions or misdirected outcomes.
An AI system that acts as a supervisor/hypervisor and monitors or reports on operations for algorithmic directed non-learning systems or subordinate limited AI systems, may be constructed with man-on-the-loop control to maintain a set of rules of engagement that vary based on command decisions…such as automatic counterfire in response to massive raid or complex mixed attack raids. In short, bias is a welcome and normal feature of systems and potentially of data sets or other methods of training. Adverse bias parameters can be identified, measured, monitored and managed for supervisory and hierarchically defined systems. This can be done in real-time and supplemented by using methods of parametric stress testing to confirm the capabilities of supervisory systems and also continuously provide a check of system performance, in machine time. Command can be maintained for AI systems as a three state situation with: automatic or independent reliance on the system, man-on-the-loop or as a distributed manual control based on the operational tempo and assessed impact of operational decision or based on the severity and need for decision attribution.
Dr. Lydia Kostopoulos posted about this blog and Linked-In and suggested the community of interest comment. Great set of questions that raise more questions.
The first question that comes to mind is how do you define ‘bias’ in the realm of war fighting? Do you use the societal norms of your host nation or organization or do you tailor your ML/AI approach to the adversary? What is bias when the adversary wants to destroy your nation, your way of life, and you? Consider the Global War on Terror (GWOT) for example. Do we label the data based on what we know (demographics, culture, language, equipment, tactics, etc) about the adversary (Al Qaeda, ISIS, Boko Haram, Al Shabaab, The Taliban, Haqqani Network, etc) in order to train the models? Contrast a GWOT adversary with a near-peer nation state adversary and how we would collect and label the data and train the models. How do we control risk to friendly forces in the model if we potentially introduce constraints due to actual or perceived biases? Look forward to the discussion.
This started out well, but wound up being a social post posing as tech. Good grief, I’m not even going to answer a bias question, as I’m tempted to simply say hooray for machines, as they won’t be concerned with political correctness.
Look larger please. The concern is lethality, success, winning. As such, the jump into machine learning is the same as the jump into hiring new personnel. You have an objective you can’t do yourself and you bring in personnel to carry it out. You already ask yourself, “Are my processes and people going to carry out what I need the way I need it?” Your concern, at least under Secretary Mattis, is whether or not you succeed. Bias would only be introduced into the discussion as a possible reason for failure, IF failure was an outcome.
Now, people have bias and they lie and hide things. Machines don’t. They will give you only the bias or deception programmed into them. They can’t introduce new bias or deception to their users. We already have a mechanism to verify the outcome of our personnel and it will be easier to do with machines.
I use the comparison of machines vs personnel because I hear some talking about machine learning like they are going to push a single button, sit back in a chair and entire missions are going to play out with no further decisions or involvement required. That’s not how this works. Like with personnel, there is training, programming, communication, and of course, verification that the end result is what was desired.
Like a gun, machine learning is an extension to our human actions and intentions. It still must be aimed, fired and verified as having hit the target.
To speak to the first question, some biases we are currently willing to accept come in the form of targeted advertisements, news articles, on so on. For instance, instead of spending your time reading an entire newspaper or skimming dozens of online articles for news regarding East Asian economic policy, your apps (such as Google News, or Apple News, which employ machine learning techniques) will know from past experience to provide you with the latest ‘Abenomics’ updates, for example. In theory, this is all great. However, we also see evidence of “misbehaving” algorithms or artificial agents which essentially make choices with a skewed bias.
The spectrum of bias in machine learning applications is vast, from the Semi-Automated Business Reservations Environment, a software sponsored by American Airlines and used by travel agents, which had a habit of over-recommending American Airlines— to Microsoft’s (unintentionally?) xenophobic chatbot Tay. However, I find the problems (i.e., biases) associated with autonomous systems are due to the human influence they receive. A machine does not “want” something to be true or false, or right or wrong. A machine is not inclined to bias, as it is a machine. However, human bias exists in every stage of machine learning from the initial creation of an algorithm to what data the machine is given, to how the machine interprets the data. In fact, this summer, a team from the Czech Republic researched and analyzed 20 cognitive biases that can be baked-in to every aspect of AI. (https://arxiv.org/pdf/1804.02969.pdf) Ultimately, the study found that these biases that scientists are unintentionally programming into AI essentially render machine learning useless. Namely, because at that point we are no longer creating an AI, we are instead obfuscating our own flawed perceptions in a black box. Microsoft’s Tay underscores ALL of the biases. However, I would argue that Tay’s downfall is entirely attributed to the environment it was exposed to. As one journalist noted, “Given that the internet is often a massive garbage fire of the worst parts of humanity, it should come as no surprise that Tay began to take on those characteristics.” (https://gizmodo.com/here-are-the-microsoft-twitter-bot-s-craziest-racist-ra-1766820160)
Currently, I believe that programming bias should be avoided when possible and thoughtfully implemented when unavoidable— but I recognize this is nearly impossible to do. Do note, I say “currently” because I think it will be possible to equip AI will a set of morals and ethical constraints that far surpass human standards. Further, programming bias need not be a blatant as the biases in the Northpoint Correctional Offender Management Profiling for Alternative Sanctions (COMPAS) criminal risk assessment system, for example, which misclassified black defendants twice as often than white defendants for violent recidivism, “and white recidivists were misclassified as low risk 63.2% more often than black defendants.” Rather, programming bias can be relatively innocent, merely the result of humans thinking they are clever or intuitive, as pictured in many examples provided by the Czech study. However, regardless of where these biases fall on the scale of utterly benign to Skynet, biases as a whole make our interpretation of data unreliable.
(https://www.rand.org/content/dam/rand/pubs/research_reports/RR1700/RR1744/RAND_RR1744.pdf)
The ability to Observe, Orient, Decide, and Act faster than our enemies on the field of battle is, and always has been a key to victory. While preparing to respond to this question, I looked over several passages from Sun Tzu’s, The Art of War. Several quotes listed below I feel still ring true and further make my case about wining OODA based scenarios, and are also areas that I feel by using AI can help determine quick and sound outcomes:
• Speed is the essence of war. Take advantage of the enemy’s unpreparedness; travel by unexpected routes and strike him where he has taken no precautions.
• In war the victorious strategist only seeks battle after the victory has been won, whereas he who is destined to defeat first fights and afterwards looks for victory.
• The expert in battle seeks his victory from strategic advantage and does not demand it from his men.
• The art of war teaches us to rely not on the likelihood of the enemy’s not coming, but on our own readiness to receive him; not on the chance of his not attacking, but rather on the fact that we have made our position unassailable.
• What the ancients called a clever fighter is one who not only wins, but excels in winning with ease.
Just as President Truman had to struggle with weighing the decision of authorizing the use of the atomic bomb on Japan, he calculated that if we continued to wage a traditional war against Japan, which would have included another Normandy-type invasion, the amount of casualties on both sides would be astronomical. I feel that using this moment in history as a benchmark, even though it may be an extreme example, it is a good example of having to make a difficult decision on what needed to be done when the world is weary from war.
Furthermore, I feel that if our adversaries on the battlefield are fielding technologies like LAWS, and we are fighting an insurmountable battle with extreme losses, the difficult decision to put aside concerns of biases will be done to ensure a strategic end to the fight.
With respect to question 2, the notion and idea of algorithms have shifted over time; from the concept of computability and computable functions to empowering computing systems with the gift of ‘intelligence,’ to learning algorithms for training computer systems to learn/create useful internal models of the world. Unfortunately, algorithmic decisions are not automatically equitable just by virtue of being the products of complex processes, and the procedural consistency of algorithms is not equivalent to objectivity. Algorithms may be mathematically optimal but ethically problematic. The question of accountability is murkier when artificial agents are involved.
Ideally, biases and fallibilities in machine-learning applications will be limited, controlled, audited, and corrected for throughout their applications. From an operational perspective, as a warfighter, I would want bias to be limited as much as possible in any application because there are potential repercussions regardless of the application being used. Of course, in the case of LAWS, for example, any potential bias or algorithmic inequitability has serious consequences (like the loss of life or lives). However, a solid argument can be made that biases or ‘incorrectly behaving’ algorithms in other uses such as protection, sustainment, and intelligence gathering could have dire consequences as well. Imagine if our intelligence community was fed only biased and inequitable data about a specific population/area and potential insurgent activity in that area. Intelligence gathering in one area alone may, of course, lead us to find more relative activity there than if we were looking elsewhere. The mere act of not surveying more areas equitably, we could find more activity in that area just by the nature of additional monitoring that region. In this case, we could potentially miss other activities and intelligence that may endanger other troops or assets. The downstream adverse effect could be just as dire as having bias or algorithmic problems in other lethal applications. If we “allow” some more bias in one area than another, then we do not take accountability and responsibility of mitigating bias in general as much as possible. Will it ever be perfect? Likely not. We are humans. We have our own inherent biases. It is not likely that we are going to be able to completely “reprogram” ourselves at this point. An algorithm is a different story. There is where we have an opportunity to correct, as much as we can, a problem of bias and fallibility that we know exists through various methods such as algorithmic transparency, auditing, and addressing ethical and biased issues in personnel developing A.I algorithms. To even partially ignore bias, or accept it more in some applications and not others, I think would be irresponsible and duplicitous.
I think that the biases in machine learning programming and algorithms will be more apparent than the inherent biases of humans-in-the-loop largely due to the social learning obstacles involved in AI learning. (Osaba, 18) “The second angle of the algorithmic bias problem often applies when working with policy or social questions. This is the difficulty of defining ground truth or identifying robust guiding principles. Our ground truth or criteria for judging correctness are often culturally or socially informed.”
If an AI system cannot clearly solve a problem or properly interpret a situation because of social/policy factor this failure will more than likely be clear to us. Whereas a human can react to the error based on the feedback (often verbal and nonverbal in speech) in a social environment AI machines may not be able to pick up on the nuances of negative responses being received. The social norms I am speaking about are often unique among varies segments of our population and further fragmented within smaller subculture groups. This becomes an issue in machine learning that relies on analysis of a large amount of data to improve. (Osaba, 19) “Machine learning algorithms have issues handling sample-size disparities. This is a direct consequence of the fact that machine learning algorithms are inherently statistical methods and are therefore subject to the statistical sample-size laws. Learning algorithms may have difficulty capturing specific cultural effects when the population is strongly segmented.” I think that the smaller size disparities in social or policy dilemmas would make the AI versus human biases more apparent.
The inherent way we as humans learn is another point I would make about visible biases being more pronounced in AI than humans. We learn our social biases throughout the course of our lives and throughout the various stages of our development incrementally this allows us to identify them, accept or deny them, change or evolve our viewpoints or even outright abandon a bias. This is not the same for an AI System. (Allen, 2011) “Why has it proven so difficult for AI researchers to build human-like intelligence, even at a small scale? One answer involves the basic scientific framework that AI researchers use. As humans grow from infants to adults, they begin by acquiring a general knowledge about the world, and then continuously augment and refine this general knowledge with specific knowledge about different areas and contexts. AI researchers have typically tried to do the opposite: they have built systems with deep knowledge of narrow areas and tried to create a more general capability by combining these systems.”
I believe the perceived biases will clearly identify an AI System as just that an artificial system without the ability to interact socially, use appropriate policy considerations or understand why we fear the machine itself leading to a resistance to trust them.
Works Cited:
Paul G. Allen “Paul Allen: The Singularity Isn’t Near.” MIT Technology Review, MIT Technology Review, 8 Jan. 2016, https://www.technologyreview.com/s/425733/paul-allen-the-singularity-isnt-near/ (Links to an external site.)Links to an external site.
Osonde A. Osaba and William Welser IV, “An Intelligence in our Image: The Risks of Bias and Errors in Artificial Intelligence,” RAND (2017) https://www.rand.org/content/dam/rand/pubs/research_reports/RR1700/RR1744/RAND_RR1744.pdf (Links to an external site.)Links to an external site.
AI is crucial to the military. Any flaws in AI, especially in the military, would hurt the nation. Prominent bias flaws in our AI systems would be mortifying for our nation. The article states that the biases in the systems do come from somewhere, “But data generation is often a social phenomenon (e.g., social media interactions, online political discourse) inflected with human biases.” It is crucial for humans not to allow biases to these algorithms. The advantage that we have is that we create these algorithms and it’s up to us to be wary of the system’s decisions. Keith Kirkpatrick highlights a riveting point in Battling Algorithmic Bias when he states, “algorithms simply grind out their results, and it is up to humans to review and address how that data is presented to users, to ensure the proper context and application of that data,”. Once again, if the data is analyzed accurately, the biases would gradually diminish. I believe that there must be a form to determine if the systems do have bias. When those biases are determined, they must be terminated. An article An Intelligence in Our Image cites examples of programming bias that is distinctive towards races. The problem with such a bias may not be so significant depending on where it is. Once the bias is determining someone’s future life, that causes a dilemma.
Osoba, Osonde, and William Welser. An Intelligence in Our Image. PDF. Santa Monica, CA: RAND Corporation, 2017.
Kirkpatrick, Keith. “Battling Algorithmic Bias.” Battling Algorithmic Bias. October 01, 2016.
https://cacm.acm.org/magazines/2016/10/207759-battling-algorithmic-bias/abstract.
Predictions that affect people’s lives is an example of how detrimental machine learning bias could be. To predict what someone will say next is one thing, but to predict if another country will attack the United States with a nuclear weapon is another. The Third Offset Strategy applies predictions as one of the offsets. The Third Offset Strategy consists of deep learning systems, which is used for indications and warnings and cyber defense. If the system is bias, how can its indications and warnings be reliable? The deep learning systems are also capable of performing electronic warfare attacks and large missile raids. The danger that such a system can cause if it contains bias is astronomical. There are elevated chances of machine learning systems predicting something wrong, take warfare for example. Predictions from bias in machine learning highlight an important concern: traditionally, success in machine learning has revolved around prediction accuracy. Yet, studies show that the algorithm that best approximates the decisions of a biased system is probably not the best algorithm for a just society. It is useful for the United States to protect and defend the nation, as well as it is useful to be capable of predicting its adversaries next move, which leads to how crucial it is for the Department of Defense to mitigate the risks.
To answer question #2, types of systems we might accept an algorithmic bias or automation bias tend to be in quickly changing, big data and quick response environments. A system along the lines of an autopilot choosing a route and flying based on fuel efficiency, time and weather for a flight. In this case we heavily rely on the choices and options the software presents us without humans challenging it with too much depth. Of course, in this sense, human interaction is still heavily required in actually deciding along with every other sense of flying the aircraft. However, a change in weather patterns that the pilots may not be aware about will allow them to utilize this data to alter the route. In this sense, we accept these bias’s because time and time again, they have been tested and proven to work with incredibly high accuracy.
Machine learning applications in non-lethal warfighting functions like sustainment, protection and intelligence should in fact be given more leeway with regards to bias. I think this is due to the fact that human lives are not typically at stake. Although incidents do happen, it is typically isolated and local. Even with machine learning, humans are still overlooking the entire operation allowing for proper intervention if needed. However, because of the high efficiency results and great benefits offered it is given more leeway with regards to bias. This is all due to the fact that the results are heavily affected by the data going in. If we trust in the data going in, we will have a bias of always trusting the algorithmic output from that dataset. This being paired with a low risk of human harm allows for a major upside and high trust.
Another instance would in which bias and leeway to the bias ended poorly was in regard to the Semi-Automated Business Reservations Environment (SABRE). SABRE was an algorithmic system that utilized airline flights and routing information to automatically display representatives with flight choices for customers. However, its “information sorting behavior took advantage of a typical user behavior to create a systematic anticompetitive bias for its sponsor.” (Osaba and Welser, 8) Typically, because we trust in the data going in, we trust in the data coming out. Thankfully, antitrust proceedings made SABRE more transparent no longer allowing for bias behavior. In regard to military and the adoption of machine learning applications assisting in a non-lethal role, more leeway is granted with regards to bias as we progress, develop and advance our military.
In response to question #1:
Since we know that algorithmic bias exists, we must be willing to accept them to some degree. This is not unlike the phenomenon of the human bias. Although we don’t always like to admit it, we all carry some bias. It should be noted here that not all bias in humans is bad. Bias carried by humans is what allows us to excel at certain tasks; they are assumptions forged by experiential learning. Bias becomes an issue in humans it when it lacks cognition or remains unchallenged, thus promoting false concepts about people, objects, or events, leading ultimately to inaccurate judgements. Thus, the same can be said for machines. If a machine were capable of checking their conclusions for accuracy or performing some kind of self-audit on its decisions, we might be more apt to accept subtle biases in programming. Yet this issue is further complicated by the inability of a machine to possess its own morality. As Osaba and Welser put it in their work An Intelligence in Our Image: The Risk of Bias and Errors in Artificial Intelligence; “there can be no meaningful morality associated with artificial agents; their behavior is casually determined by human specification” (Osaba and Welser IV, 7). Morality, according to the authors, is that which is characterized by choice and empathy. While autonomous machines powered by algorithmic learning appear to possess the power of choice, they are more closely executing educated decisions. This issue strikes at the concept of algorithmic adaption in response to input; the ‘data diet’ (and/or level of data exposure) upon which an autonomous machine operates can seriously impact the decisions it is likely to make. It is also why human biases are present in machine learning systems. Even if we attempt to hide them, or restrict so-called ‘sensitive’ data sets (gender, race, sexuality, nationality), machine learning algorithms develop similar bias in their absence.
So what do we accept? How much are we, the humans allegedly ‘removed’ from the loop, willing to tolerate in our autonomous systems? For one, I believe the answer to this question changes based upon the degree to which popular bias held by humans negatively impacts your life. For example, various case studies describe the racial discrimination demonstrated by artificial agents employed to conduct various justice system related functions. Since such artificial agents demonstrated bias towards racial minorities, I’d be willing to bet racial minorities are less likely to accept those biases in programming. Thus, this answer is dependent upon sociocultural-demographic characteristics. With respect to Lethal Autonomous Weapons Systems, I believe there is certainly a line drawn in the sand. Examples of commonly accepted bias in programming can be found in airfare websites which advertise certain companies (who happen to be stakeholders) over others, or in suggestive search functions (mainly for their common accuracy). While we don’t love that these programming biases exist, we tolerate them for the perceived benefits they provide. Areas where we do not tolerate programming bias are those which lead to a violation of our fundamental rights. Employability or criminality scores, which infringe upon privacy, due process, and equal opportunity legislation, are examples of areas in which we perceive the benefits of well-instructed machines as less valuable than the ability of human reason. Unfortunately, due to the ‘data diet,’ machine learning algorithms become a representation of that which is available to them for consumption. Biased input data equates to biased outputs. The absence of human reason in these scenarios is what makes them particularly frightening in my opinion. We cannot accept the possibility (though it may be inevitable) of programming bias in LAWS, on both moral, ethical, and legal grounds. Based upon what we know about the vulnerability paradox associated with machine learning, LAWS might demonstrate programming bias with particularly devastating consequences. Thus, we can see with relative clarity what we are willing to tolerate.
It is important that we recognize that there will be biases in machine learning programming and algorithms. Once we recognize this, we can look at things objectively and analyze from there. Though humans are biased, I think that machine biases can remain steady and not waiver like those of humans. Though this might be a negative, I think that it makes it easier when identifying the bias and knowing that it is there. Some types of biases, according to a study done at Carnegie Mello University, are training data bias, algorithmic focus bias, algorithmic processing bias, transfer context bias, and interpretation bias (Danks).
First, we must identify the bias in the system. It needs to be decided if a bias is actually a problem or not. If a bias is considered negative, then the options of changing the algorithm or taking the bias out need to be thought of. Sometimes an unbiased algorithm is unachievable and we must use the one with the least bias (Danks). With biases being a part of machine learning applications, they can be hard to trust all the time- or certain people that are targeted may be unwilling to accept the use of these applications. I think that we should use them carefully and be sure to recognize and address any bias in the program. As Osoba and Welser said, “While
human decision making is also rife with comparable biases that artificial agents might exhibit, the question of accountability is murkier when artificial agents are involved” (Osoba), though humans are also biased, at least they are easier to hold accountable than machines.
Danks, David, and Alex John London. Algorithmic Bias in Autonomous Systems. PDF. Pittsburgh: Carnegie Mellon University, 2017.
Osoba, Osonde, and William Welser. An Intelligence In Our Image. PDF. Santa Monica: RAND Corporation, 2017.