(Editor’s Note: The Mad Scientist Laboratory is pleased to present a companion piece to last Thursday’s post that addressed human-machine networks and their cross-domain effects. On 10 January 2018, CAPT George Galdorisi, (U.S. Navy-Ret.), presented his Mad Scientist Speaker Series topic entitled, Designing Unmanned Systems For the Multi-Domain Battle. CAPT Galdorisi has distilled the essence of this well-received presentation into the following guest blog post — enjoy!)
The U.S. military no longer enjoys technological superiority over a wide-range of potential adversaries. In the words of former Deputy Secretary of Defense Robert Work, “Our forces face the very real possibility of arriving in a future combat theater and finding themselves facing an arsenal of advanced, disruptive technologies that could turn our previous technological advantage on its head — where our armed forces no longer have uncontested theater access or unfettered operational freedom of maneuver.”
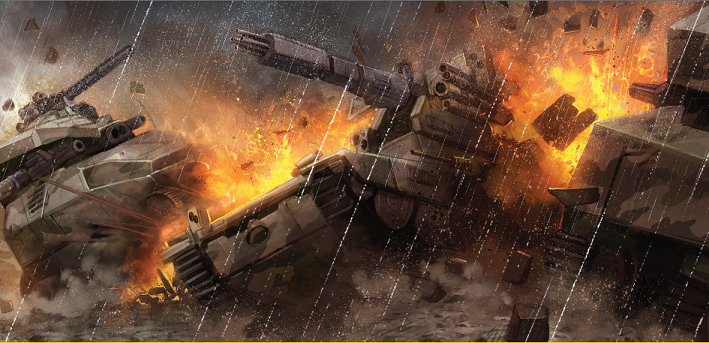
Illustration: Don Hudson & Kinsun Lo • Brought to you by Army Cyber Institute at West Point
The Army Cyber Institute’s graphic novel, Silent Ruin, posits one such scenario.
In order to regain this technological edge, the Department of Defense has crafted a Third Offset Strategy and a Defense Innovation Initiative, designed to help the U.S. military regain technological superiority. At the core of this effort are artificial intelligence, machine learning, and unmanned systems.
Much has been written about efforts to make U.S. military unmanned systems more autonomous in order to fully leverage their capabilities. But unlike some potential adversaries, the United States is not likely to deploy fully autonomous machines. An operator will be in the loop. If this is the case, how might the U.S. military best exploit the promise offered by unmanned systems?
One answer may well be to provide “augmented intelligence” to the warfighter. Fielding unmanned vehicles that enable operators to teach these systems how to perform desired tasks is the first important step in this effort. This will lead directly to the kind of human-machine collaboration that transitions the “artificial” nature of what the autonomous system does into an “augmented” capability for the military operator.
But this generalized explanation begs the question — what would augmented intelligence look like to the military operator? What tasks does the warfighter want the unmanned system to perform to enable the Soldier, Sailor, Airman, or Marine in the fight to make the right decision quickly in stressful situations where mission accomplishment must be balanced against unintended consequences?
Consider the case of an unmanned system conducting a surveillance mission. Today, an operator receives streaming video of what the unmanned system sees, and in the case of aerial unmanned systems, often in real-time. But this requires the operator to stare at this video for hours on end (the endurance of the U.S. Navy’s MQ-4C Triton is thirty hours). This concept of operations is an enormous drain on human resources, often with little to show for the effort.
Using basic augmented intelligence techniques, the MQ-4C can be trained to deliver only that which is interesting and useful to its human partner. For example, a Triton operating at cruise speed, flying between San Francisco and Tokyo, would cover the five-thousand-plus miles in approximately fifteen hours. Rather than send fifteen hours of generally uninteresting video as it flies over mostly empty ocean, the MQ-4C could be trained to only send the video of each ship it encounters, thereby greatly compressing human workload.
Taken to the next level, the Triton could do its own analysis of each contact to flag it for possible interest. For example, if a ship is operating in a known shipping lane, has filed a journey plan with the proper maritime authorities, and is providing an AIS (Automatic Identification System) signal; it is likely worthy of only passing attention by the operator, and the Triton will flag it accordingly. If, however, it does not meet these criteria (say, for example, the vessel makes an abrupt course change that takes it well outside normal shipping channels), the operator would be alerted immediately.
For lethal military unmanned systems, the bar is higher for what the operator must know before authorizing the unmanned warfighting partner to fire a weapon — or as is often the case — recommending that higher authority authorize lethal action. Take the case of military operators managing an ongoing series of unmanned aerial systems flights that have been watching a terrorist and waiting for higher authority to give the authorization to take out the threat using an air-to-surface missile fired from that UAS.
Using augmented intelligence, the operator can train the unmanned aerial system to anticipate what questions higher authority will ask prior to giving the authorization to fire, and provide, if not a point solution, at least a percentage probability or confidence level to questions such as:
• What is level of confidence this person is the intended target?
• What is this confidence based on?
– Facial recognition
– Voice recognition
– Pattern of behavior
– Association with certain individuals
– Proximity of known family members
– Proximity of known cohorts
• What is the potential for collateral damage to?
– Family members
– Known cohorts
– Unknown persons
• What are the potential impacts of waiting versus striking now?
These considerations represent only a subset of the kind of issues operators must train their unmanned systems armed with lethal weapons to deal with. Far from ceding lethal authority to unmanned systems, providing these systems with augmented intelligence and leveraging their ability to operate inside the enemy’s OODA loop, as well as ours, enables these systems to free the human operator from having to make real-time (and often on-the-fly-decisions) in the stress of combat.
Designing this kind of augmented intelligence into unmanned systems from the outset will ultimately enable them to be effective partners for their military operators.
If you enjoyed this post, please note the following Mad Scientist events:
– Our friends at Small Wars Journal are publishing the first five selected Soldier 2050 Call for Ideas papers during the week of 19-23 February 2018 (one each day) on their Mad Scientist page.
– Mark on your calendar the next Mad Scientist Speaker Series, entitled “A Mad Scientist’s Lab for Bio-Convergence Research,” presented by Drs. Cooke and Mezzacappa, from RDECOM-ARDEC Tactical Behavior Research Laboratory (TBRL), scheduled for 27 February 2018 at 1300-1400 EST.
– Headquarters, U.S. Army Training and Doctrine Command (TRADOC) is co-sponsoring the Bio Convergence and Soldier 2050 Conference with SRI International at Menlo Park, California, on 08-09 March 2018. This conference will be live-streamed; click here to watch the proceedings, starting at 0840 PST / 1140 EST on 08 March 2018.
CAPT George Galdorisi, (U.S. Navy–Ret.), is Director for Strategic Assessments and Technical Futures at SPAWAR Systems Center Pacific. Prior to joining SSC Pacific, he completed a 30-year career as a naval aviator, culminating in fourteen years of consecutive service as executive officer, commanding officer, commodore, and chief of staff.
The fundamental issue I have with current AI/ML discussion (as applied to the military context) is that we tend to use the terminology indiscriminately. We mix simple, relatively well understood, ML techniques (such as image recognition) with more abstract “Strong” AI properties that are currently not even proven to be achievable. This makes it hard to differentiate fact from fiction, or from near term realistic application of the technology to more far term hypotheticals. We need to do a better job delineating between what AI/ML learning is today and how it can be applied Vs what we want AI to be able to do in the future and the implications thereof.
For additional information on AI Trends, please see: https://madsciblog.tradoc.army.mil/11-artificial-intelligence-ai-trends/