[Editor’s Note: Mad Scientist Laboratory is pleased to review Prediction Machines: The Simple Economics of Artificial Intelligence by Ajay Agrawal, Joshua Gans, and Avi Goldfarb, Harvard Business Review Press, 17 April 2018. While economics is not a perfect analog to warfare, this book will enhance our readers’ understanding of narrow Artificial Intelligence (AI) and its tremendous potential to change the character of future warfare by disrupting human-centered battlefield rhythms and facilitating combat at machine speed.]
This insightful book by economists Ajay Agrawal, Joshua Gans, and Avi Goldfarb penetrates the hype often associated with AI by describing its base functions and roles and providing the economic framework for its future applications. Of particular interest is their perspective of AI entities as prediction machines. In simplifying and de-mything our understanding of AI and Machine Learning (ML) as prediction tools, akin to computers being nothing more than extremely powerful mathematics machines, the authors effectively describe the economic impacts that these prediction machines will have in the future.
The book addresses the three categories of data underpinning AI / ML:
Training: This is the Big Data that trains the underlying AI algorithms in the first place. Generally, the bigger and most robust the data set is, the more effective the AI’s predictive capability will be. Activities such as driving (with millions of iterations every day) and online commerce (with similar large numbers of transactions) in defined environments lend themselves to efficient AI applications.
Input: This is the data that the AI will be taking in, either from purposeful, active injects or passively from the environment around it. Again, defined environments are far easier to cope with in this regard.
Feedback: This data comes from either manual inputs by users and developers or from AI understanding what effects took place from its previous applications. While often overlooked, this data is critical to iteratively enhancing and refining the AI’s performance as well as identifying biases and askew decision-making. AI is not a static, one-off product; much like software, it must be continually updated, either through injects or learning.
The authors explore narrow AI rather than a general, super, or “strong” AI. Proclaimed Mad Scientist Paul Scharre and Michael Horowitz define narrow AI as follows:
“… their expertise is confined to a single domain, as opposed to hypothetical future “general” AI systems that could apply expertise more broadly. Machines – at least for now – lack the general-purpose reasoning that humans use to flexibly perform a range of tasks: making coffee one minute, then taking a phone call from work, then putting on a toddler’s shoes and putting her in the car for school.” – from Artificial Intelligence What Every Policymaker Needs to Know, Center for New American Security, 19 June 2018
These narrow AI applications could have significant implications for U.S. Armed Forces personnel, force structure, operations, and processes. While economics is not a direct analogy to warfare, there are a number of aspects that can be distilled into the following ramifications:
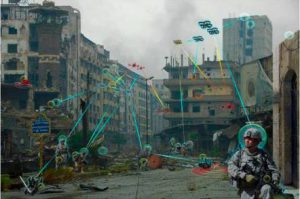
1. The battlefield is dynamic and has innumerable variables that have great potential to mischaracterize the ground truth with limited, purposely subverted, or “dirty” input data. Additionally, the relative short duration of battles and battlefield activities means that AI would not receive consistent, plentiful, and defined data, similar to what it would receive in civilian transportation and economic applications.
2. The U.S. military will not be able to just “throw AI on it” and achieve effective results. The effective application of AI will require a disciplined and comprehensive review of all warfighting functions to determine where AI can best augment and enhance our current Soldier-centric capabilities (i.e., identify those workflows and processes – Intelligence and Targeting Cycles – that can be enhanced with the application of AI). Leaders will also have to assess where AI can replace Soldiers in workflows and organizational architecture, and whether AI necessitates the discarding or major restructuring of either. Note that Goldman-Sachs is in the process of conducting this type of self-evaluation right now.
3. Due to its incredible “thirst” for Big Data, AI/ML will necessitate tradeoffs between security and privacy (the former likely being more important to the military) and quantity and quality of data.
4. In the near to mid-term future, AI/ML will not replace Leaders, Soldiers, and Analysts, but will allow them to focus on the big issues (i.e., “the fight”) by freeing them from the resource-intensive (i.e., time and manpower) mundane and rote tasks of data crunching, possibly facilitating the reallocation of manpower to growing need areas in data management, machine training, and AI translation.
This book is a must-read for those interested in obtaining a down-to-earth assessment on the state of narrow AI and its potential applications to both economics and warfare.
If you enjoyed this review, please also read the following Mad Scientist Laboratory blog posts:
Takeaways Learned about the Future of the AI Battlefield
Leveraging Artificial Intelligence and Machine Learning to Meet Warfighter Needs
… and watch the following presentations from the Mad Scientist Robotics, AI, and Autonomy – Visioning Multi-Domain Battle in 2030-2050 Conference, 7-8 March 2017, co-sponsored by Georgia Tech Research Institute:
Artificial Intelligence and Machine Learning: Potential Application in Defense Today and Tomorrow,” presented by Mr. Louis Maziotta, Armament Research, Development, and Engineering Center (ARDEC).
Unmanned and Autonomous Systems, presented by Paul Scharre, CNAS.